mAbLab: A Novel Solution
Quick Look
The Method Behind the Madness: Transformer Architecture
mAbLab employs a transformer-based architecture, a machine-learning framework that uses an encoder-decoder method to make multimodal decisions. Using self-attention, the model can create its own rules for decision-making that can address the nuances of the data at hand. Moreover, transformers in a mAb context are highly accurate in producing proteins that optimize 3D structure and binding affinity, allowing them to create comparatively advantageous products compared to traditional methods.
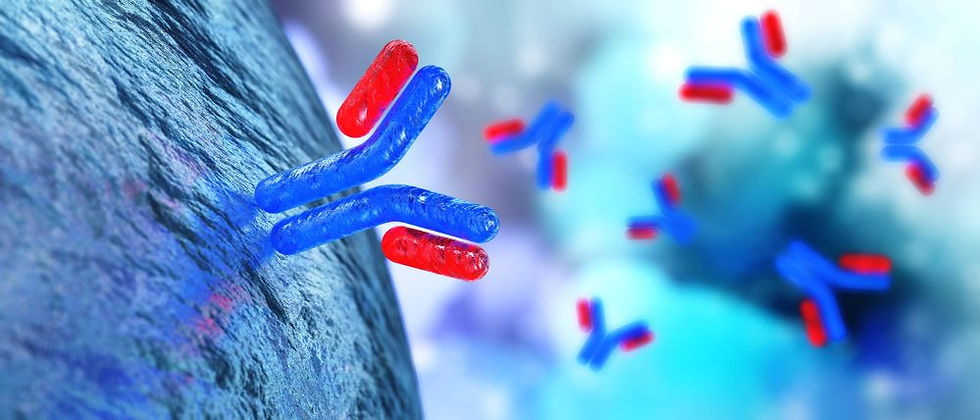
The Step by Step
mAbLab uses a positive-feedback loop, meaning whenever its model makes predictions, it is fed back in to improve accuracy of future predictions.
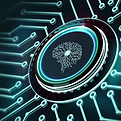
Data Compilation
Using pre-existing data from sources like the IEDB and NCBI, data needs to be standardized, including information like the amino acid sequence of epitopes, CDRH (binding) sites, antigens, as well as information about conformation like binding affinity and linear representation of a mAb's 3D structure.
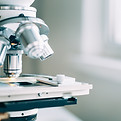
Predictions
Using mAbLab, we can input any protein sequence—gated channels, RTKs, GPCRs, whatever it may be—and find a mAb the binds to specific functional sites as an antagonist, agonist, etc.
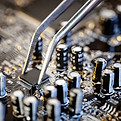
Model Dev & Training
mAbLab runs off a transformer specifically designed for biological use-cases. Using either preexisting models or creation of one's own, training has to occur that looks at nuances in biological data.
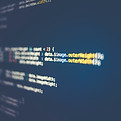
In Silico Analysis
Using an in silico docking simulators, we can validate mAbLab produced mAbs in silico before wasting unnecessary resources to do several in vitro tests.
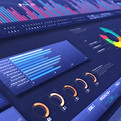
Testing & Fine-Tuning
Once mAbLab is built and trained, we can move on to in vitro testing. Using molecular level imaging tools such as FRET and functionality tests such as analyzing ion channel activity, we can validate mAbLab’s accuracy and industry utility. Additionally, we can finetune the transformer to specific types of antibodies such as T cell α and β chains, dimeric IgA, and pentameric IgM.
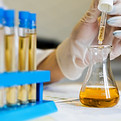
Manufacturing & Transplantation
With the predicted mAb amino acid sequence, we can use large scale protein generation optimizers such as ICOR to convert the amino acid sequence to a DNA sequence. From here, we can use restriction endonucleases such as CRISPR Cas9 to engineer a plasmid to carry the mAb gene. This plasmid can then be inserted into bacteria through methods such as electroporation which gives us a mAb producing factory.

Looking to the Future: Breakthroughs and Next Steps
The effectiveness of transformer technologies in a variety of use-cases ensures its validity when synthesized with development of pharmaceutical products. By shifting the R&D paradigm in the mAb industry, mAbLab seeks to incite change throughout the entire drug discovery-and-design process as a whole, facilitating an influx of more efficient, stronger, and cheaper therapeutics to battle incoming disease.
Complex Etiology
The complex nature of certain cancers makes it so that simple language-processing software can’t address intricate biological mechanisms behind pathology. As a result, more research has to be done to create a ML-based model specifically for such biological predictions.
Adaptability
The process of manually inserting mAbs into one’s bloodstream can have adverse effects if the antibody is rejected and acts as a self-antigen. As a result, expansion of in-silico frameworks to test these products is necessary to truly speed up lead times.
Interpretability
The self attention nature of transformers leaves scientists in the dark about the rules underlying predicted mAbs. As a result, research into "supervised-transformers," one that can take feedback from an external source to influence decision making, may be benficial.